Dealing with the effects of sensor displacement in wearable activity recognition
- PMID: 24915181
- PMCID: PMC4118358
- DOI: 10.3390/s140609995
Dealing with the effects of sensor displacement in wearable activity recognition
Abstract
Most wearable activity recognition systems assume a predefined sensor deployment that remains unchanged during runtime. However, this assumption does not reflect real-life conditions. During the normal use of such systems, users may place the sensors in a position different from the predefined sensor placement. Also, sensors may move from their original location to a different one, due to a loose attachment. Activity recognition systems trained on activity patterns characteristic of a given sensor deployment may likely fail due to sensor displacements. In this work, we innovatively explore the effects of sensor displacement induced by both the intentional misplacement of sensors and self-placement by the user. The effects of sensor displacement are analyzed for standard activity recognition techniques, as well as for an alternate robust sensor fusion method proposed in a previous work. While classical recognition models show little tolerance to sensor displacement, the proposed method is proven to have notable capabilities to assimilate the changes introduced in the sensor position due to self-placement and provides considerable improvements for large misplacements.
Figures
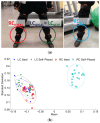
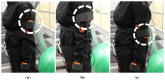
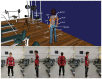
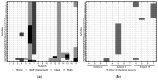
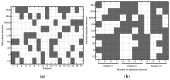
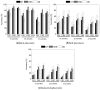
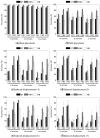
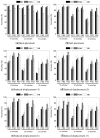
Similar articles
-
Automatic Recognition of Activities of Daily Living Utilizing Insole-Based and Wrist-Worn Wearable Sensors.IEEE J Biomed Health Inform. 2018 Jul;22(4):979-988. doi: 10.1109/JBHI.2017.2734803. Epub 2017 Aug 1. IEEE J Biomed Health Inform. 2018. PMID: 28783651
-
An incremental learning method based on probabilistic neural networks and adjustable fuzzy clustering for human activity recognition by using wearable sensors.IEEE Trans Inf Technol Biomed. 2012 Jul;16(4):691-9. doi: 10.1109/TITB.2012.2196440. Epub 2012 May 16. IEEE Trans Inf Technol Biomed. 2012. PMID: 22614724
-
Performance Evaluation of State of the Art Systems for Physical Activity Classification of Older Subjects Using Inertial Sensors in a Real Life Scenario: A Benchmark Study.Sensors (Basel). 2016 Dec 11;16(12):2105. doi: 10.3390/s16122105. Sensors (Basel). 2016. PMID: 27973434 Free PMC article.
-
A review of wearable sensors and systems with application in rehabilitation.J Neuroeng Rehabil. 2012 Apr 20;9:21. doi: 10.1186/1743-0003-9-21. J Neuroeng Rehabil. 2012. PMID: 22520559 Free PMC article. Review.
-
Motion tolerance in wearable sensors--the challenge of motion artifact.Annu Int Conf IEEE Eng Med Biol Soc. 2007;2007:1542-5. doi: 10.1109/IEMBS.2007.4352597. Annu Int Conf IEEE Eng Med Biol Soc. 2007. PMID: 18002263 Review.
Cited by
-
mDurance: A Novel Mobile Health System to Support Trunk Endurance Assessment.Sensors (Basel). 2015 Jun 5;15(6):13159-83. doi: 10.3390/s150613159. Sensors (Basel). 2015. PMID: 26057034 Free PMC article.
-
Activity Recognition Invariant to Sensor Orientation with Wearable Motion Sensors.Sensors (Basel). 2017 Aug 9;17(8):1838. doi: 10.3390/s17081838. Sensors (Basel). 2017. PMID: 28792481 Free PMC article.
-
LARa: Creating a Dataset for Human Activity Recognition in Logistics Using Semantic Attributes.Sensors (Basel). 2020 Jul 22;20(15):4083. doi: 10.3390/s20154083. Sensors (Basel). 2020. PMID: 32707928 Free PMC article.
-
Training Data Selection and Optimal Sensor Placement for Deep-Learning-Based Sparse Inertial Sensor Human Posture Reconstruction.Entropy (Basel). 2021 May 10;23(5):588. doi: 10.3390/e23050588. Entropy (Basel). 2021. PMID: 34068635 Free PMC article.
-
PhysioDroid: combining wearable health sensors and mobile devices for a ubiquitous, continuous, and personal monitoring.ScientificWorldJournal. 2014;2014:490824. doi: 10.1155/2014/490824. Epub 2014 Sep 10. ScientificWorldJournal. 2014. PMID: 25295301 Free PMC article.
References
-
- Ermes M., Parkka J., Mantyjarvi J., Korhonen I. Detection of daily activities and sports with wearable sensors in controlled and uncontrolled conditions. IEEE Trans. Inf. Technol. Biomed. 2008;12:20–26. - PubMed
-
- Stiefmeier T., Roggen D., Ogris G., Lukowicz P., Tröster G. Wearable activity tracking in car manufacturing. IEEE Pervas. Comput. 2008;7:42–50.
-
- Chernbumroong S., Atkins A., Yu H. Activity classification using a single wrist-worn accelerometer. Proceedings of the 5th International Conference on Software Knowledge Information, Industrial Management and Applications; Benevento, Italy. 8–11 Spetember 2011; 2011. pp. 1–6.
-
- Atallah L., Lo B., King R., Yang G.Z. Sensorpositioning for activity recognition using wearable accelerometers. IEEE Trans. Biomed. Circuits Syst. 2011;5:320–329. - PubMed
Publication types
MeSH terms
LinkOut - more resources
Full Text Sources
Other Literature Sources
Medical